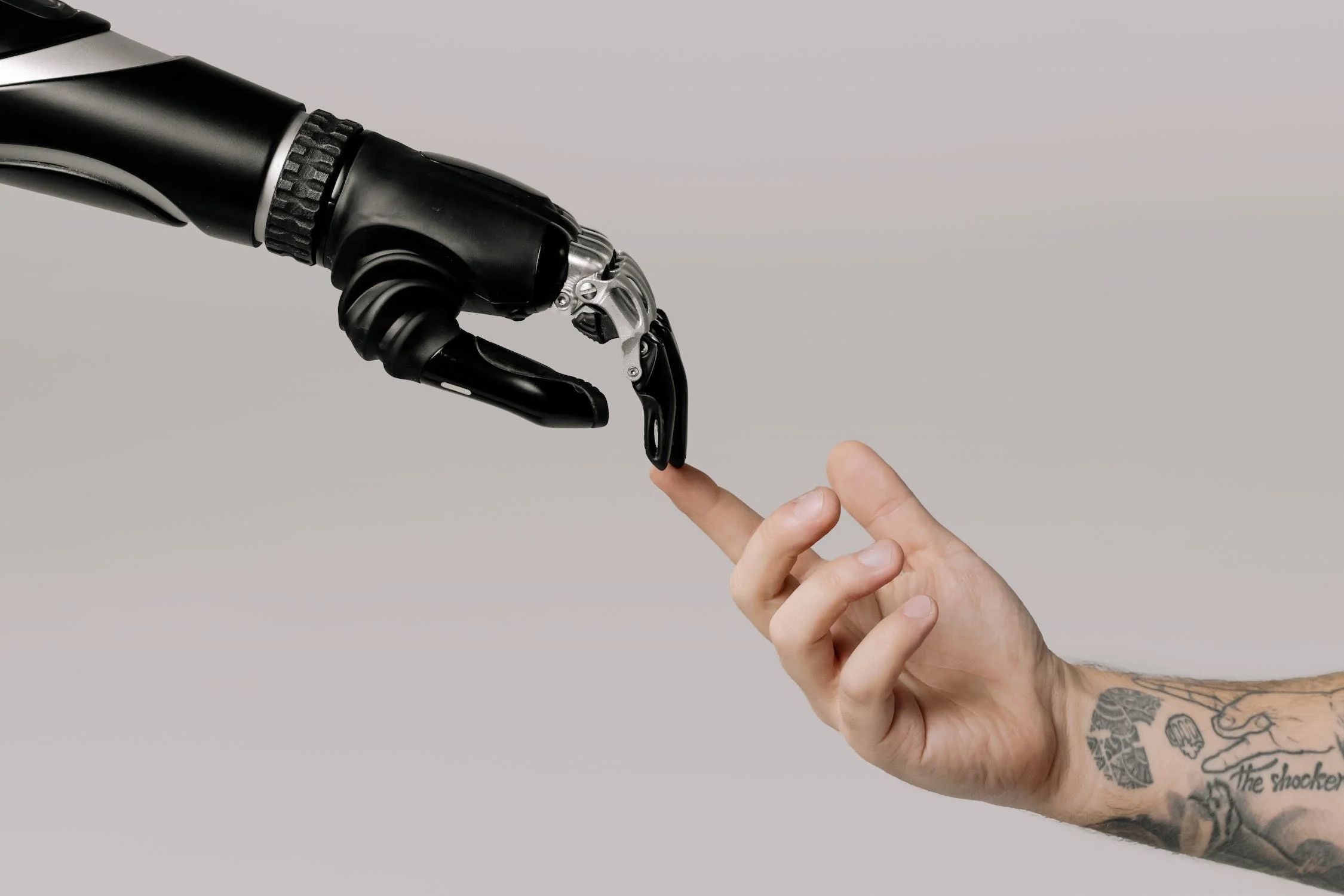
07 Jan AI VS MACHINE LEARNING: HOW DO PROS EXPLAIN THE DIFFERENCE?
Artificial intelligence (AI) and machine learning are often used interchangeably, but experts observe that the pair of terms actually refer to unique concepts. On the former front, AI is the broader idea that machines can perform tasks that mimic human intelligence, while machine learning is a specific approach to achieving smart tech capabilities that involves algorithms “learning” from data to improve at tasks over time.
As IT thought leader Andrew Ng explains, artificial intelligence is that branch of engineering and science devoted to making intelligent machines, while machine learning is the field of study within AI focused on algorithms that can learn from data to make predictions or decisions. So machine learning represents a subset of what AI aims to achieve in general.
Stanford computer scientist Fei-Fei Li describes artificial intelligence as enabling computers to think, while machine learning enables them to learn. In her view, AI is about building intelligent systems that have functionality similar to human minds – for example, the ability to see patterns, understand language, plan, and solve complex problems. Machine learning, on the other hand, gives computers the specific capacity to learn and improve at tasks without explicit programming, by examining large volumes of data for patterns.
For example, an AI system designed for autonomous vehicles would utilize various AI capabilities to perceive the road, understand visual information, plan routes, predict traffic patterns, communicate with human drivers, and ultimately drive the vehicle independently. This is only possible by integrating a range of AI functionalities. The machine learning component refers specifically to the algorithms powering the system’s pattern recognition capabilities – by analyzing driving data over time, it learns to identify roads, signs, obstacles etc. and navigate more effectively.
Yoshua Bengio, pioneer in deep learning, yet another subset within machine learning, provides further clarification. He explains that AI incorporates all methods aimed at enabling machines to function intelligently, while machine learning homes in on one such strategy of statistical pattern recognition. Deep learning then focuses specifically on neural networks for machine learning, modeled after the neuronal networks in human brains.
So in essence, observers say that AI represents the broad goal of mimicking human-level intelligence of all kinds in machines. Machine learning denotes one methodology to equip systems with the ability to learn from experience rather than explicitly programmed rules. And deep learning highlights neural networks as a singularly successful architecture under the machine learning approach, taking loose inspiration from information processing in the human mind. Together, these fields cover both the grand vision of AI and very specific tools to bring us closer to that eventuality.